|
|
|
本文已被:浏览 47次 下载 11次 |
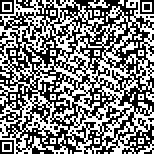 码上扫一扫! |
|
基于生物信息学结合网络药理学探讨党参改善低氧适应的作用机制 |
刘金杰1,2,3,4, 黄湘龙1,2,3,4, 宋柯妍1,2,3,4, 张俊华1,2,3,4, 李玉红1,2,3,4, 李霄1,2,3,4, 张晗1,2,3,4
|
1.天津中医药大学中医药研究院, 天津 301617;2.组分中药国家重点实验室, 天津 301617;3.现代中药创制全国重点实验室, 天津 301617;4.方剂学教育部重点实验室, 天津 301617
|
|
摘要: |
[目的] 采用机器学习结合网络药理学筛选的方法,预测中药党参促进机体低氧适应的潜在药效成分与作用机制。[方法] 收集党参活性成分及潜在作用靶点;从GEO数据库中获取低氧下差异表达基因,整合GeneCards数据库中低氧适应相关的基因,获取低氧适应和活性成分共同的靶点,构建蛋白-蛋白互作网络。随后结合2种机器学习方法筛选得到核心靶点,利用外部数据集及iHypoxia数据库进行验证,分子对接预测党参主要活性成分与核心靶点之间的结合能力。[结果] 筛选得到19个党参活性成分,有效成分作用靶点338个,低氧适应关键靶点1 642个,共同靶点66个。核心靶点主要涉及细胞周期蛋白依赖激酶1(CDK1)、缺氧诱导因子1α(HIF1A)、激酶插入域受体(KDR)、白细胞介素-6(IL-6)、趋化因子受体(CXCR4)、基质金属蛋白酶2(MMP2)、胎盘生长因子(PGF)等。GO分析得到的生物学过程主要涉及对缺氧的反应、血管生成、内皮细胞增殖、分化等方面;KEGG信号通路分析,主要包括低氧诱导因子-1(HIF-1)和磷脂酰肌醇-3-激酶(PI3K)-蛋白激酶B(Akt)信号通路。分子对接显示党参炔苷、黄豆黄素、紫丁香苷、党参碱和党参苷Ⅰ等活性成分和关键靶点之间具有较好的结合活性。[结论] 党参具有提高机体低氧适应能力的作用,并可能与调控HIF-1信号通路及HIF1A、KDR和CKD1等靶点相关。 |
关键词: 低氧适应 党参 网络药理学 GEO数据库 机器学习 分子对接 |
DOI:10.11656/j.issn.1672-1519.2025.02.12 |
分类号:R285.5 |
基金项目:国家重点研发计划项目(2022YFC3501800);天津市科技计划项目(23ZYJDSS00020);天津中医药大学新教师科研启动项目(XJS2022209)。 |
|
Exploring the mechanism by which Codonopsis Radix improves hypoxia adaptation based on bioinformatics and network pharmacology |
LIU Jinjie1,2,3,4, HUANG Xianglong1,2,3,4, SONG Keyan1,2,3,4, ZHANG Junhua1,2,3,4, LI Yuhong1,2,3,4, LI Xiao1,2,3,4, ZHANG Han1,2,3,4
|
1.Institute of Traditional Chinese Medicine, Tianjin University of Traditional Chinese Medicine, Tianjin 301617, China;2.State Key Laboratory of Component-Based Chinese Medicine, Tianjin 301617, China;3.National Key Laboratory of Chinese Medicine Modernization, Tianjin 301617, China;4.Key Laboratory of Pharmacology of Traditional Chinese Medical Formulae, Ministry of Education, Tianjin 301617, China
|
Abstract: |
[Objective] To predict the key targets and the potential active ingredients of Codonopsis Radix on improving hypoxia adaptation using a combination of machine learning and network pharmacology screening methods. [Methods] The active components and potential targets of Codonopsis Radix were collected. Differentially expressed genes under hypoxia were obtained from the GEO database,and genes related to hypoxia adaptation were integrated from the GeneCards database. Common targets of hypoxia adaptation and active components were identified by constructing a protein-protein interaction network. Two machine learning methods were used to screen for core targets,which were validated using external datasets and the iHypoxia database. Molecular docking was used to predict the binding capacity between the main active components of Codonopsis Radix and the core targets. [Results] AA total of 19 active components of Codonopsis Radix were identified,with 338 active component targets,1 642 key targets for hypoxia adaptation,and 66 common targets. The core targets mainly involved CDK1,HIF1A,KDR,IL6,CXCR4,MMP2 and PGF. GO analysis revealed that the main biological processes were related to the response to hypoxia,angiogenesis,endothelial cell proliferation,and differentiation. KEGG pathway analysis identified 20 related signaling pathways,including the HIF-1 and PI3K-Akt pathways. Molecular docking showed that the active components Lobetyolin,Glycitein,Syringin,Codonopsine,and Tangshenoside I had good binding activity with key targets. [Conclusion] Codonopsis Radix may enhance the hypoxia adaptation ability of the human body by regulating the HIF-1 signaling pathway and its interaction with targets such as HIF1A,KDR,and CDK1. |
Key words: hypoxia adaptation Codonopsis Radix network pharmacology GEO database machine learning molecular docking |
|
|
|
|